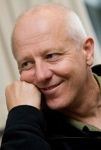
Richard Lockhart, Professor
Department of Statistics and Actuarial Science
|Phone: (778) 782 3264 | Messages: (778) 782 3803 | Fax: (778) 782 4368
Office: K 10561 | Email: lockhart (at) sfu (dot) ca
-
Cambridge Mini-course, Lent, 2017
Course Notes, 8 Mar
Inference for High Dimensional Regression
Lecture Slides -
Big Data Workshop at SFU
PIMS page
Statistical Inference for Large Scale Data
Program, abstracts -
Big Data Workshop at UBC
PIMS page
Big Data in Environmental Science
Program, abstracts -
Statistics Canada
The Daily -
United States Census Bureau
Data -
UK Statistics
StatsUserNet -
Putnam solutions
1985 1986 1987 1988 1989 -
The Statistical Society of Canada
Home -
Physics and Statistics at BIRS
July 2010 Workshop -
My old home page
I used to have a simpler page -
SFU sites
My department
My university
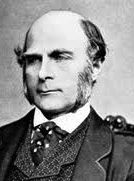
Galton-Watson-Bienayme processes
Sir Frances Galton was a cousin of Charles Darwin and an eminent scientist. He is responsible for the idea of ``regression to the mean'' which now gives the name for the statistical methods of regression analysis. Galton worked with Watson to describe the process of extinction of family names -- working in a world where sons inherited familiy names from fathers and women changed their names on marriage. The model then supposes that generation 0 is a single man. In generation 1 there are Z_1 sons of the first man generated from some ``offspring'' distribution $p(k)=P(Z=k)$. Independently of each other each of these sons has $Z_{2,i}$ sons of his own for $i=1,...,Z_1$, again according to the distribution $p$. We actually observe only $Z_2=\sum_i Z_{2,i}$. In the third generation second generation male number $i$ generates $Z_{3,i}$ offspring independently of all others and so on.
In other words generation number $n+1$ has total size $Z_{n+1}$ which is a sum of $Z_n$ independent and identically distributed numbers of sons $Z_{n+1,i}$; each of these has distribution $p$. I have worked on inference in these processes with Peter Guttorp . Peter has written a great book about inference problems in these (and more general) processes: Statistical inference for branching processes, Wiley, 1991.
My own role with Peter was in investigating inference for $p$.
The results we have here
are mostly negative, showing the non-existence of consistent estimates
of parameters of the offspring distribution other than the mean and
variance. We have looked at the maximum likelihood estimate
of the variance of the offspring distribution. Though this offspring
distribution is not consistently estimable its mean and variance are.
It is known that mle of the mean is consistent and we think we can
prove that the mle of the variance is also consistent. Our proof
relies on a uniform approximation of the likelihood based on a
uniform version of the local central limit theorem.
Lockhart, R. A. (1982). On the non-existence of consistent estimates in
Galton-Watson processes. J. Appl. Prob., 19 842--846.
Draft paper
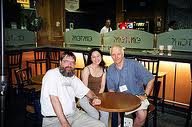
Sparsely sampled random walks
I like to work on large sample theory with what I think of as clean, simple conditions. Peter Guttorp and I did this
sort of thing in work on inference in irregularly sampled random walks. That work was motivated by a paper I wrote applying the
local central limit theorem to prove that on an explosive trajectory of a Bienaym\'e-Galton-Watson only the mean, variance and
perhaps one other arithmetic parameter admit consistent estimates.
Guttorp, P. and Lockhart, R.A. (1989). Estimation in sparsely
sampled random walks. Stoch. Proc. Their Appl., 31 315--320.